ISYE 6420: Bayesian Statistics
Instructional Team
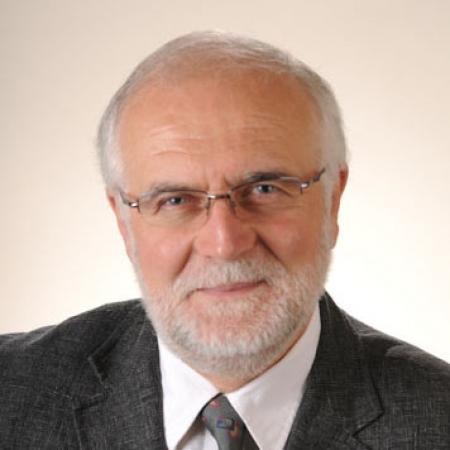
Brani Vidakovic
Creator, Instructor
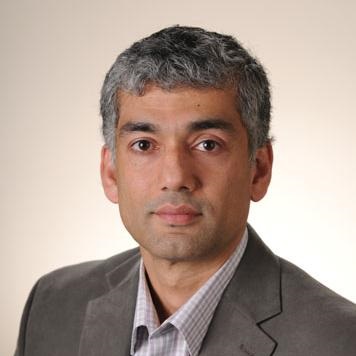
Roshan Joseph
Instructor
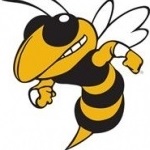
Aaron Reding
Head TA
Overview
This course provides an introduction to Bayesian statistical inference and its applications.
More information is available on the ISYE 6420 course website.
Course Goals
By the end of this course, students will model and infer from Bayesian philosophical perspective. The aim is to make you proficient in the following:
- Given a real-life data set, to select an appropriate statistical model to conduct inference, to formulate any prior information in terms of probability distributions (priors), and to understand what the conducted inference implies.
- In addition to understanding concepts and being able to select the right methodology for the problem in hand, the course is aimed at hands on approaches and delivering explicit results.
- Another aim of this course is for you to build a solid basis for your data modeling skills, so you can continue to learn throughout your career. New techniques will certainly be developed after you graduate, and we want you to be able to pick them up quickly.
- In addition, when you accumulate more information about the problem in hand, you will be able to coherently incorporate this information and update your inference.
The core of Bayesian approach to data modeling is Markov Chain Monte Carlo method. Although you would be exposed to theoretical concepts of MCMC and several step-by-step examples will be discussed, we will not cover the details of mathematics and algorithms under the hood, or deeper mastery of the modeling needed to set up an efficient MCMC chain.
We will rely on the expert system provided by WinBUGS/OpenBUGS software.
Sample Syllabus
Spring 2025 syllabus (PDF)
Fall 2024 syllabus (PDF)
Spring 2023 syllabus (PDF)
Note: Sample syllabi are provided for informational purposes only. For the most up-to-date information, consult the official course documentation.
Before Taking This Class...
Suggested Background Knowledge
Students should have taken introductory courses in probability in statistics. They should also have basic programming proficiency and be comfortable with calculus.
Technical Requirements and Software
- High-speed internet connection
- Laptop or desktop computer with a minimum of a 2 GHz processor and 2 GB of RAM
- Windows for PC computer or Mac iOS for Apple computers
Academic Integrity
All Georgia Tech students are expected to uphold the Georgia Tech Academic Honor Code. This course may impose additional academic integrity stipulations; consult the official course documentation for more information.